MONDAY, 13 MARCH 2017
Since the ancient Greeks wrote the great automaton, Talos of Crete, into myth, science fiction has tinkered much with artificial intelligence (AI) in its well stocked playground. Isaac Asimov is perhaps the most famous man-handler of sci-fi’s best beloved toy, his three laws of robotics proving highly influential. Subsequently, lm has dissolved AI’s delicious possibilities and dangers into the mainstream, with 2001: A Space Odyssey’s apathetic Hal perhaps proving the most famous fictional AI in the world, the eerie scene in which it faces a reboot adding emotional colour to the debate on AI and personhood. Move into more recent years, and AI still provides a fertile spawning ground for the screenwriter. 2013 brought us Her, a film that explored the romantic relationship between a man and his disembodied operating system, Samantha, whose mind evolved and expanded way beyond human ken, tangling with thousands of lovers. 2015 saw a film about the ultimate Turing test, Ex Machina, in which a psychopathic tech bro creates an embodied, alluringly human and also rather psychopathic AI, Ava, by designing it to learn organically, getting Ava’s ‘brain’ to ‘rearrange on a molecular level’. 2015 also yielded the film Chappie, the titular character of which progresses into personhood by growing, mentally, from a toddler to a teenager before it begins to conceive of morality and human emotion. The film adroitly appreciates that achieving true intelligence is a developmental process, so why should it not be the same for robots?
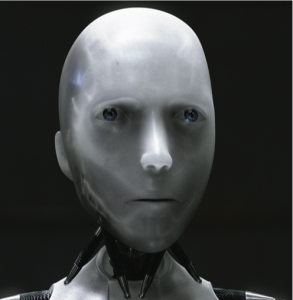
Sonny: Can you?
Image: I,Robot
It is clear that the type of intelligence writers are envisioning here is quite different to what we mean by intelligence in everyday parlance. Intuitively, one might expect that tasks humans find easy, for example identifying objects, moving our arms and conveying meaning through language, might also be easy to program in machines, unlike tasks that humans find difficult, such as beating a grandmaster at chess. However, Charles Babbage’s 1849 analog general computing engine gave the first clear demonstration that number churning was going to be a relatively easy task, while the victory of IBM’s Deep Blue over then reigning chess grandmaster Gary Kasparov in 1997 showed that computers can operate within a framework of rules to super-human levels.
Disembodiment
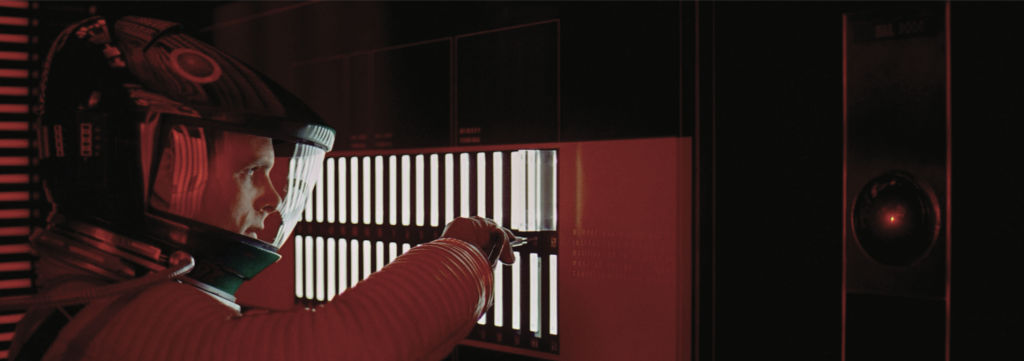
Mind and matter are often completely uncoupled in computer science and robotics. ough initially inspired by the brain, writing AI algorithms has very little to do with brain cells (other than the employment of the programmer’s own grey matter!). The first AI systems therefore substituted biology for pure human cleverness.
All of us interact with AIs on an almost daily basis, although not ones with human intelligence. A good example are e-mail filters. Spam filters perform an intelligent function insofar as they employ machine learning to keep away instant millionaire schemes, Russian women looking for kind Western husbands, and Nigerian Princes. Broadly, they do this by examining the e-mails that you, the user, designate as spam or not-spam, and break down their content into key features that can then be used to automatically filter incoming e-mail.
This breed of AI, that does not try to mimic a real human brain, has proven incredibly commercially successful. The 1990s saw it applied to many specific tasks, from those in logistics to medical diagnosis; tasks in which value can be obtained from sifting through huge amounts of data intelligently. This is has allowed us to make great leaps in virtual personal assistants, leading to the development of Siri, Alexa and Cortana, and the death of the Microsoft paper clip thing.
These assistants creep a little closer to a general artificial intelligence. In the case of these ‘narrow AI’, like the spam filter, if the rules of the game were suddenly to change rather quickly – you have been chosen to get £250 million into your HSBC account to help a Wall Street banker in trouble instead of the usual exiled prince, a scheme that most humans would instantly recognise as spam even if known keywords are absent – the AI will initially get the wrong answer. Although a learning algorithm may subsequently send the baloney peddlers to your spam folder if you filter the first few manually, it has not taken the general sense of what a spam e-mail is, and applied this general understanding to new situations.A common approach has been to attempt to reduce human intelligence to a symbolic representation, and from this build something like an artificial general intelligence. A symbolic representation is one that can be translated and made understandable by a human, and many of the AI programmes that employ them follow, essentially, “if this, then this is this – if this, then do that” rules.
The success of these approaches in the mid 1990s in developing AI systems that could answer geography questions posed naturally by humans, suggest chemical structures to organic chemists and diagnose infectious blood bourne diseases, eclipsed fledgling bio-inspired fields like cybernetics.Ultimately, incorporating greater generality like this has seen the evolution of Microsoft’s paperclip into Microsoft’s Cortana, and may yet see it through to something like Her’s Samantha. Fuelled by Moore’s law, the theoretical two-yearly doubling in the computing power of a chip, these AIs could be extremely computationally powerful, as well as empathetically savvy.However sheer human cleverness cannot completely circumvent the aegis of biology. e general computation of the nervous system in learning from experience and transforming sensory input into motor output is di cult for smart-bots to achieve in an organically intelligent way with purely symbolic AI. So maybe we should move from a system where we can write and understand all the rules, even if the results surprise us, to one which is ‘sub- symbolic’, like Ava, whose operation cannot be read by humans, and can be as much of an enigma as the human brain itself.
Artificial Neural Networks
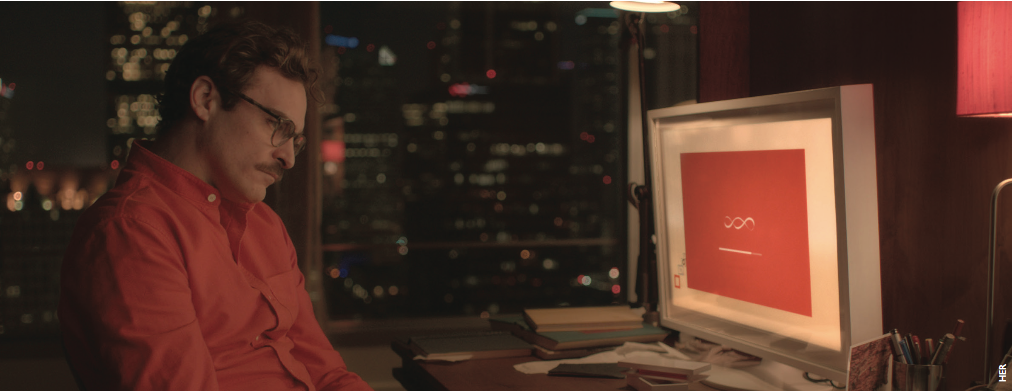
Artificial neural networks are connected arrays of computing units, usually implemented in software on conventional computers. They were originally simplified mathematical descriptions of real, biological neurons – the computing units of the brain, spinal cord and sensory and motor inlets and outlets of organismal nervous systems. Originally models of neural function were simple because we could not model more complex things. Individual ‘neurons’ are often modelled as units that can either be on, and influencing connected neurons to which they output, or off , depending on the degree of input from their incoming connectors. This ignores many realities of biological neuronal function: the various types of stimulation one neuron can receive from another, how the morphology of a neuron changes the way it works, how they ‘fire’ in ‘spikes’ of activity with different patterns over space and time, how their connections change, etc.
Adding some of these details can make our arti cial networks better. It can make them learn
by changing how they connect in response to environmental cues. Donald Hebb’s learning rule, which effectively states that neurons that “ wire together wire together”, is the most commonly used method, strengthening connections between linked neurons that activate simultaneously. Learning can also be made to occur by reinforcement, with ‘rewards’ and ‘punishments’ given to a network for performing well or badly.
The study of artificial neural networks is essentially split by two interconnected streams: “how does the nervous system work” and, “how can we make machines that are intelligent?” While neither problem is solved their tributaries have been extensively studied, yielding fruit from driverless cars on one end of the comp-bio spectrum to models of clinical neuropathologies on the other. One end has thrived o of getting AI programmes to do things that are useful (and biological accuracy be damned!), while the other has gleaned much from looking at the electrical properties of real, individual neurons, and applying the toolkit of molecular genetics. Somewhere in the middle live the once rare but increasingly populous species of academic, the connectionists, who attempt to draw wiring diagrams of real nervous systems or cognitive processes and ultimately, see if they can make them run in silicon.
Even strikingly simple neural structures can do seemingly incredible things. A ‘perceptron’, which in its simplest form is a single artificial neuron with two inputs, can distinguish between two simple scenarios – choose the pill blue or red? John Hop eld’s interconnected, symmetric wheel of neurons can actually store ‘associative memories’. For every pattern of neurons a user activates in the wheel, a new and unique pattern can be yielded or completed – that is essentially what your brain has done when I mentioned blue and red bills, it yielded an associated pattern of letters, The Matrix. Hopefully.
But much more can be done. Deep learning is a neural network AI approach that uses many layers of simulated neurons whose connection strengths change with experience, so that activation patterns that result from tens to potentially millions of neurons talking to one another in the network are adaptable. However, between input and output, the network’s behaviour is unclear – a black box system.
They can do some pretty impressive things, even if we cannot always see how. Google’s DeepMind algorithm’s victory over a world champion at the ancient Chinese game of Go actually involved something akin to intuition since unlike chess the number of possible states of a Go board outnumbers the atoms in the known universe.
The DeepMind algorithm reached its prowess by generalising and truly strategising. Unfortunately though, it cannot generalise what it has learned in the Go environment, for example it cannot verbally tell us about or conceive of what it has learned. Therefore it does not really demonstrate true cognition. Lee Se-Dol, the current human world champion, does, and commented at a press conference before his defeat “I believe human intuition and human senses are too advanced for artificial intelligence to catch up. I doubt how far [DeepMind] can mimic such things”. Here, human intuition failed to intuit intuitiveness of computer intuition. Tough, admittedly, unlike the algorithm, Lee Se-Dol did not get to play himself 30 million times before the match, a fact that really highlights the computational difference between human and artificial intelligence.
So, how do we get a cleverer neural net, especially when we are not that sure how it
actually works? Well, how did biology do it? e human brain was carved by impassive millennia
of evolution. We can improve artificial neural networks in the same way, quite literally evolving them by natural selection, taking the best bits of competing algorithms, and passing them on to
new generations that continue to compete with one another. A bit of developmental biology can even be thrown into the mix, with some researchers claiming better performance if they allow neural networks to literally ‘grow’ – branching out their connections and ‘dividing’ like real cells over time, based on the activity level of each unit.
However, while complex reasoning and language processing have been demonstrated by a handful of artificial neural network to date, true cognition has not. Moreover, even artificial neural networks require a lot of programming and electricity. Chappie and Ava both had an actual body, and the chips that allow us to put AI ‘minds’ into robotic ‘bodies’ are starting to come up against fundamental performance limits. Moore’s law is bending closer to its asymptote. In 2012 Google developed a piece of AI that recognised cats in videos, without ever explicitly being told what a cat was, but it took 16,000 processors to pull off.
Neuromorphism
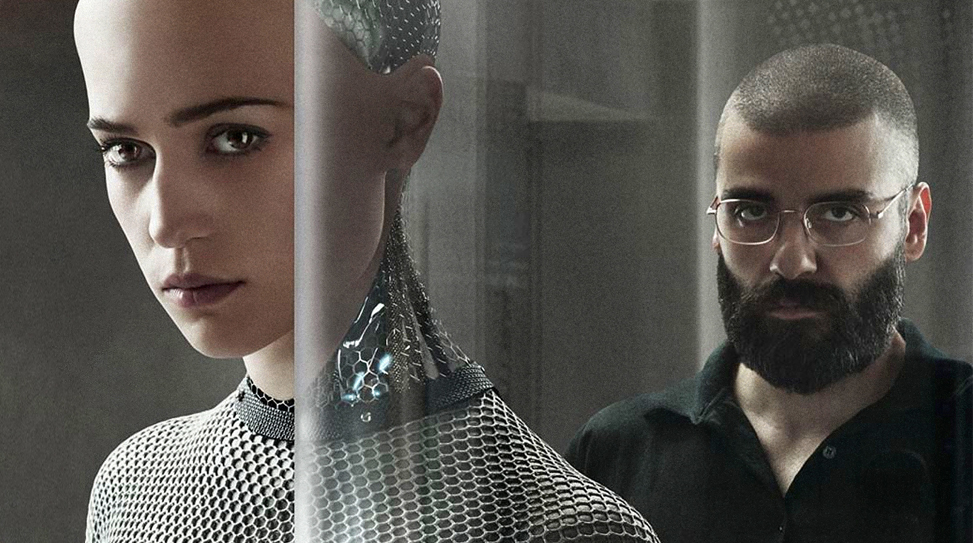
While not completely untrue of conventional computation, biological neural networks have to deal with strong resource limits. Problems of resource delivery and allocation, space constraints and energy conservation are all much weaker for artificial neural networks, for which we can just add more computers. These limits have undoubtedly determined much of the physical layout, and therefore logic, of real nervous systems. Real neurons are also analog, with a continuous output scale, not digital and so binary like modern computers. Therefore, in many ways their actual function more closely resembles the plumbing in your kitchen than the Mac in your study.
Modern computers are ‘von Neumann machines’, meaning that they shuttle data between a central processor and their core memory chips, an architecture more suited to number crunching than brain simulation. Neuromorphic engineering is an emerging eld that attempts to embed arti cial neural networks in unorthodox computing hardware that combine storage and processing. ese neurons, like biological ones, communicate to each other in ‘spikes’ and the ‘strength’ of their activity is denoted by the frequency of these spikes.
As Demiurge, a Swiss-based start-up that aims to build AI based on neuromorphic engineering, puts it on their amboyant website “deep learning is a charted island in the vast uncharted waters of bio- inspired neural networks. e race of discovery in arti cial intelligence only starts when we sail away from the island and deep into the ocean.” Demiurge has received £6.65 million in Angel investment to try to develop a neuromorphic AI system, which they plan to ‘raise’ like an infant animal. They
claim that “the blindness of deep learning and the naiveness of [learning from reward/punishment] prohibit” both approaches from generating basic consciousness, which they see as enabling “spatiotemporal pattern recognition and action selection”. They want the system to learn consciously, like Chappie, effectively growing up from a baby into something analogous to personhood.
The Blue Brain Project, however, aims to simulate bits of real brain. Just pause and think how hard
it is to tackle such a problem. Here, you have a dense block of tissue, where, in the case of the rat cortex for example, approximately 31,000 neurons can be packed into a volume the size of a grain of sand. In a tour de force study last year in Nature, Henry Markram and colleagues simulated this very brain grain based on twenty years of accumulated, extremely detailed data. Unfortunately, despite being the largest effort of its kind, the simulation did not glean anything completely novel about the function of neural microcircuits. “A good model doesn’t introduce complexity for complexity’s sake,” Chris Eliasmith at the University of Waterloo noted to Nature.
Instead of Markram’s ‘bottom-up’ approach Eliasmith has tried to lower himself down into
the biological abyss from on high. He makes use of ‘semantic pointer architecture,’ the hypothesis that patterns of activity amongst groups of neurons impress some semantic context, and that an assembly of these ensembles compose a shifting pattern with a speci c meaning. e interplay between these patterns is what he claims underlies cognition. It is all more than a little abstract – but then again thinking back to that tiny chunk of brain meat, how could it not be? A 2012 study in Science used this principle to move a robotic arm to provide answers to complex problems assessed through a digital eye, using 2.5 million artificial neurons to do so. Markram was a little dismissive at the time: “It is not a brain model”. Fair enough, the attempt was not grounded in real neuroanatomy, but it had managed to build an AI from biological principles. Eliasmith has since added some bottom- up detail and concluded that greater detail did not improve his AI’s performance in this case, just increased the computational cost.
Modern technology has allowed us to gain much from grains of brain. e burgeoning connectionist eld has been using genetic techniques to highlight speci c neural circuits with uorescent jelly sh proteins, and electrical techniques to see which brain areas in uence which, and in so doing, try to draw up interaction webs. Doing this at extremely high resolution is, however, very difficult. Only one entire nervous system has ever been fully reconstructed at a sufficient resolution to account for all connections between all neurons, that of the ‘worm’, Caenorhabditis elegans. It took over ten years to fully trace the connections of the mere 302 neurons in this animal, and now five years in a second effort is half way through finding all neural connections in a vinegar fly larva, which has contains 10,000 neurons. The human brain has about 86 billion neurons. Just to store image data capturing all these neurons at an appropriate resolution would take about a zettabyte. In real terms that is approximately 1,000 data centres of crème de la crème Backblaze data storage pods, which, stacked into four stories, would take up about the same area as central Cambridge.
So, while theoretically simulating a real brain would create a brain, we can only attempt it with much abstraction. As Peter Dayan at the Gatsby in London has put it to Nature, “the best kind of modelling is going top-down and bottom-up simultaneously”. In the AI world, this is more or less what neuromorphic engineering does. The way robots learn about the world, taking advantage of many sources of sensory input and unravelling it with their neuromorphic chips, could inform the next stage of smartphone devices, just as the current generation was bio-inspired. Future chips may enable smartphones to instantly recognise people you know in your photos, anticipate who you will meet in the day and automatically photograph people who walk into your kitchen only if they steal your coco pops.
In reality, the AI of the future can hope to take cues from both neuromorphic engineering and von Neumann machines, being something that can think like a human and a computer. Now that is something that would be terrifyingly useful.
The advent and end of thinking
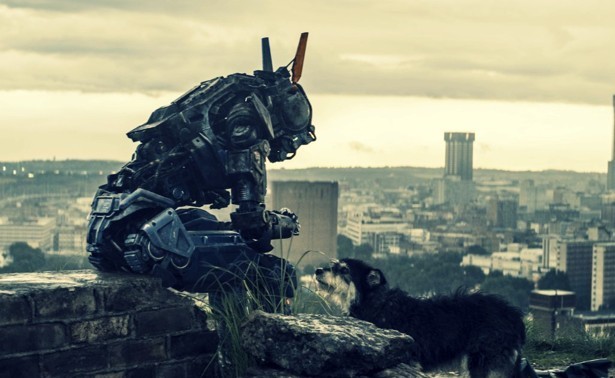
As with any new technology, there are many concerns surrounding AI. Irving Good, who worked with Alan Turing in Bletchley Park, noted in 1965 that “the first ultra-intelligent machine is the last invention that man need ever make”, because from then on mankind’s thinking can be outsourced. Should this technological singularity be reached, however, the dangers are highly unlikely to deliver a Matrix-type situation. But it is something that very much worries people, including lauded physicist Stephen Hawking and tech entrepreneur Elon Musk, who signed an open letter against military AI research, calling it an ‘existential threat’. A commonly envisioned scenario is one in which such an AI does everything in its power to prevent being shut down so that it can continue improving its functionality (Hal: “Dave, stop. Stop, will you? Stop, Dave. Will you stop Dave? Stop, Dave.”). Interestingly, the reaction of the CEO of Demiurge, Idonae Lovetrue, was to put out an advert to hire a science fiction writer. Why? She told BlueSci that “science fiction is one of the least invasive and most effective simulators to study and shape the multidimensional implications of AI technologies...it is crucial to thoroughly and responsibly test both [AI] technology via robotic simulators and its implications via such social simulators...”